Analyzing noisy, high-dimensional gene expression data
Most of our knowledge about gene regulatory networks has been obtained largely from perturbation experiments that vary e.g. environmental conditions or genotype. We developed an alternative approach that harnesses the power of high-throughput gene expression measurements (RNAseq) to extract functional relationships from the standing expression variation across individuals within a population. Using both single-cell and whole-animal RNA sequencing data, we demonstrate how a rich set of co-regulated gene modules can be uncovered from transcriptomic variability of individuals within unperturbed populations. To robustly extract interpretable clusters from the strong noise background, we devised a novel, versatile clustering approach based on network theory and the statistical physics of percolation on random geometric graphs. With a foundation in the generic behavior of random networks near their percolation critical point, our method is broadly applicable, beyond gene expression, to any noisy, high-dimensional data that sample variation across individuals within a population.
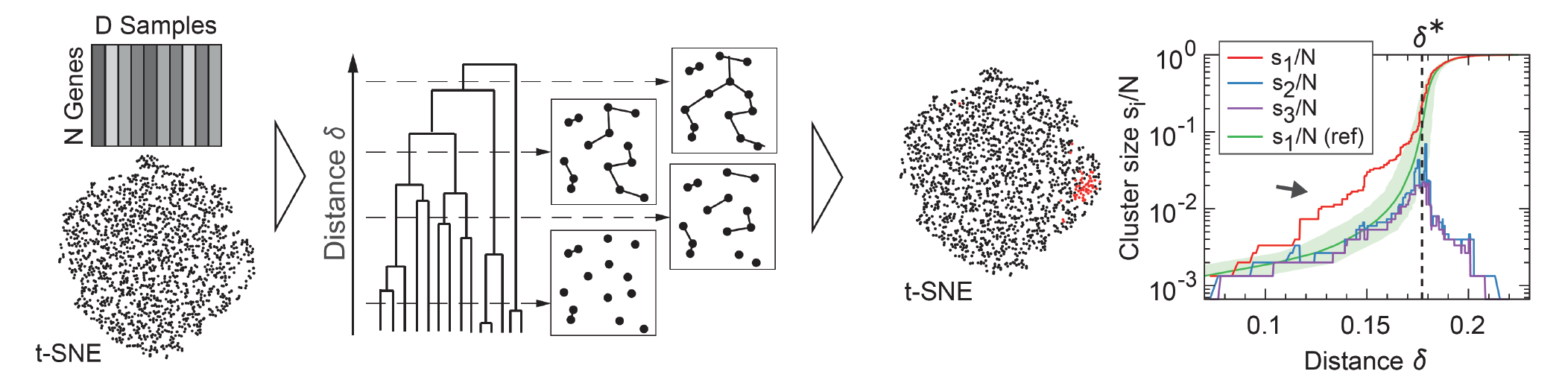
References
- Werner, S., Rozemuller, W.M., Ebbing, A., Alemany, A., Traets, J.J.H, van Zon, J.S., van Oudenaarden, A., Korswagen, H.C., Stephens, G.J. & Shimizu, T.S. (2020). Functional modules from variable genes: Leveraging percolation to analyze noisy, high-dimensional data. bioRxiv. https://doi.org/10.1101/2020.06.10.143743